The Noise Problem
Background noise which is usually present in DX (distant) communication over amateur radio is challenging and creates listening fatigue. The noise is like listening to an AM radio station in a distant city fading in and out of the static. Amateur radio noise reduction has gone through multiple generations of technology to reduce noise, but eliminating noise has been elusive. Each new generation of noise reduction technology creates a significant demand from operators to upgrade their radios. Individuals who participate in amateur radio contests spend a lot on the components of their radio station to make as many DX contacts as possible during a contest. Contesters would have an easier time to score points with noise elimination. Noise also contributes to some operators’ focus on local communication since it typically utilizes noise-free and high-fidelity FM modulation which is easy to hear.
The AI Claim
I was skeptical when I read “Pretty awesome #AI noise canceling” from TheOperator on X (Twitter) earlier this week. I decided to try it out. It only took 10 minutes to configure the “RM Noise” Windows program and virtually connect it to my HF FlexRadio “server” with SmartSDR DAX. The process was easy, including registering my call sign with RM Noise.
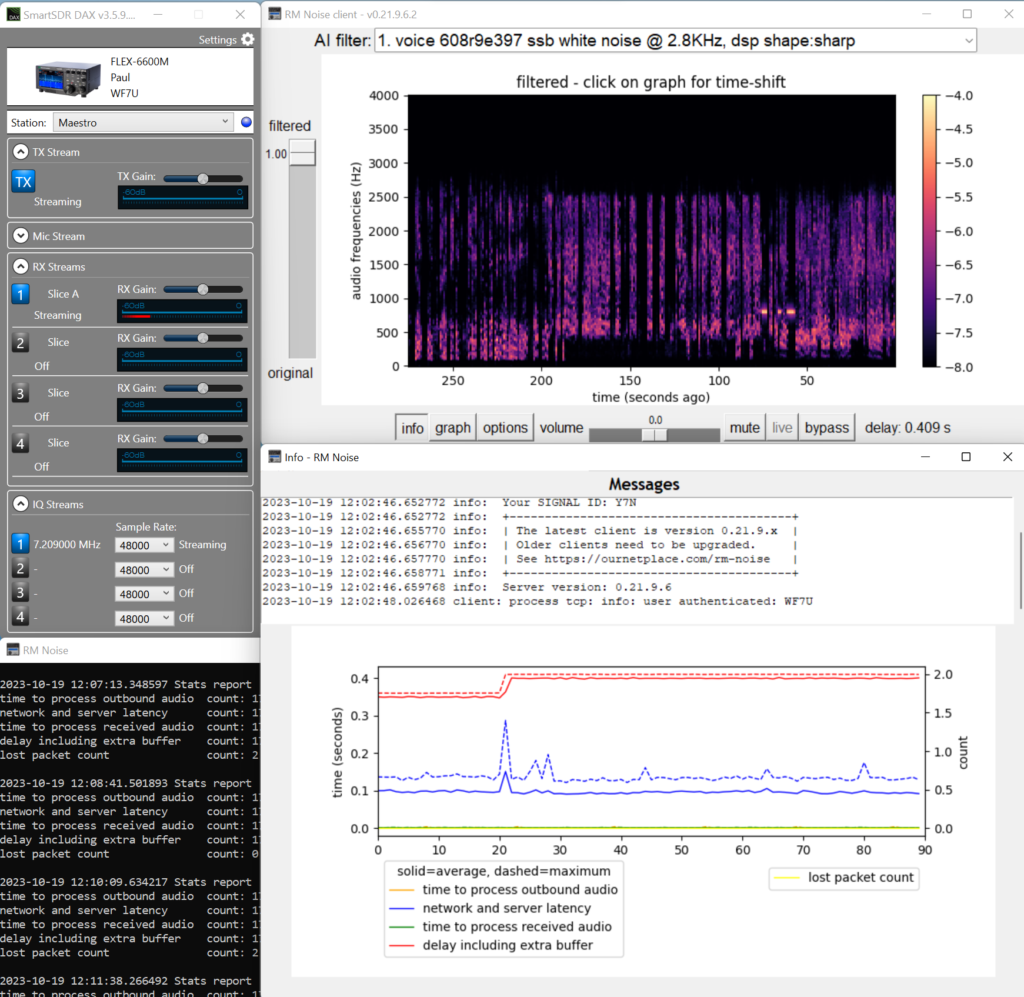
FlexRadio is a state-of-the-art Software Defined Radio (SDR) – the Cadillac of HF amateur radios. It cost more than the first used car I bought. It’s not much more challenging to connect RM Noise to other HF Amateur Radios since the popularity of WSJT digital modulation uses the same radio interface.
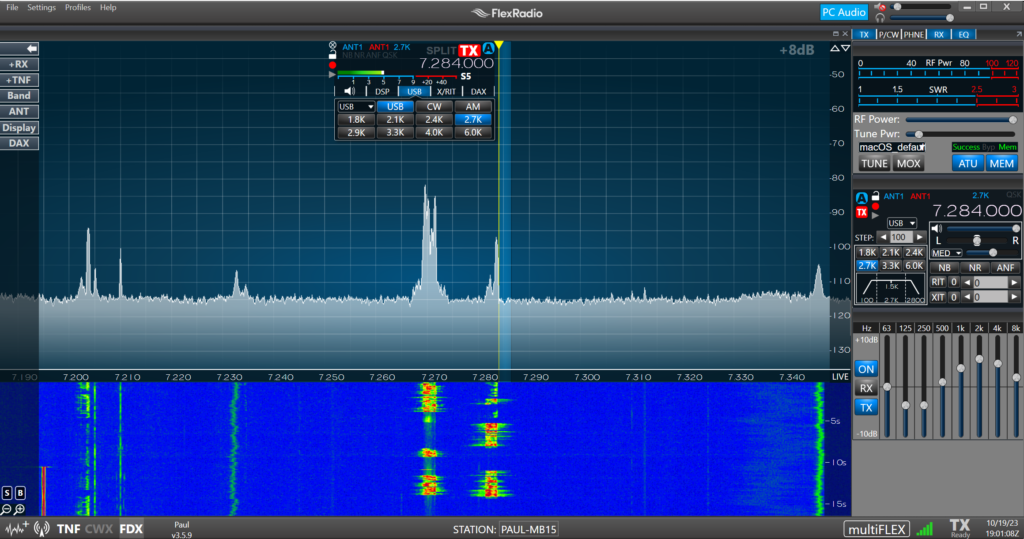
How Did it Work?
I was shocked listening to a net (amateur radio meeting) using RM Noise. Noise was eliminated without buying a new radio. I could hear a conversation with a weak signal barely above the noise floor that was absent on the pan adapter. I now believe a comment I read stating that they can no longer tolerate their radio without it.
Today was the first time I listened to my HF radio all day. Typically, I would get tired from the noise after an hour. I didn’t experience any noise fatigue. The sound quality is similar to listening to a local AM radio station. This is surprising since the dominant single-sideband (SSB) communication on HF rarely sounds as good as AM modulation.
I already found that RM Noise is indispensable. There are opportunities to improve it further: Weak signals are quiet. Your radio’s automatic level control (ALC) doesn’t “work” after RM Noise filtering. My FlexRadio ALC keeps a consistent audio level, which may include a lot of noise. With the loud noise component removed, only a quieter and understandable audio signal is left. Filtering adjacent radio signals must be performed on the radio itself since the software doesn’t include this functionality.
Now I understand Jensen Huang’s words from earlier this year, “AI is at an inflection point, setting up for broad adoption reaching into every industry.” , i.e., including Amateur Radio.
How does it work & the importance of AI model customization
I’ve previously experimented with NVIDIA Broadcast, which runs on my local PC with an NVIDIA RTX 3080. The 3080 includes AI functionality. Broadcast provided similar results to RM Noise for strong signals. Broadcast offered limited value since the weaker signals were dropped as noise. The difference between Broadcast and RM Noise is AI model customization. RM Noise was trained with a customized noise reduction model with the traffic heard on amateur radio phone (voice) and CW (morse code) traffic. If NVIDIA created an “Amateur Radio Noise removal” effect, Broadcast could work the same as RM Noise.
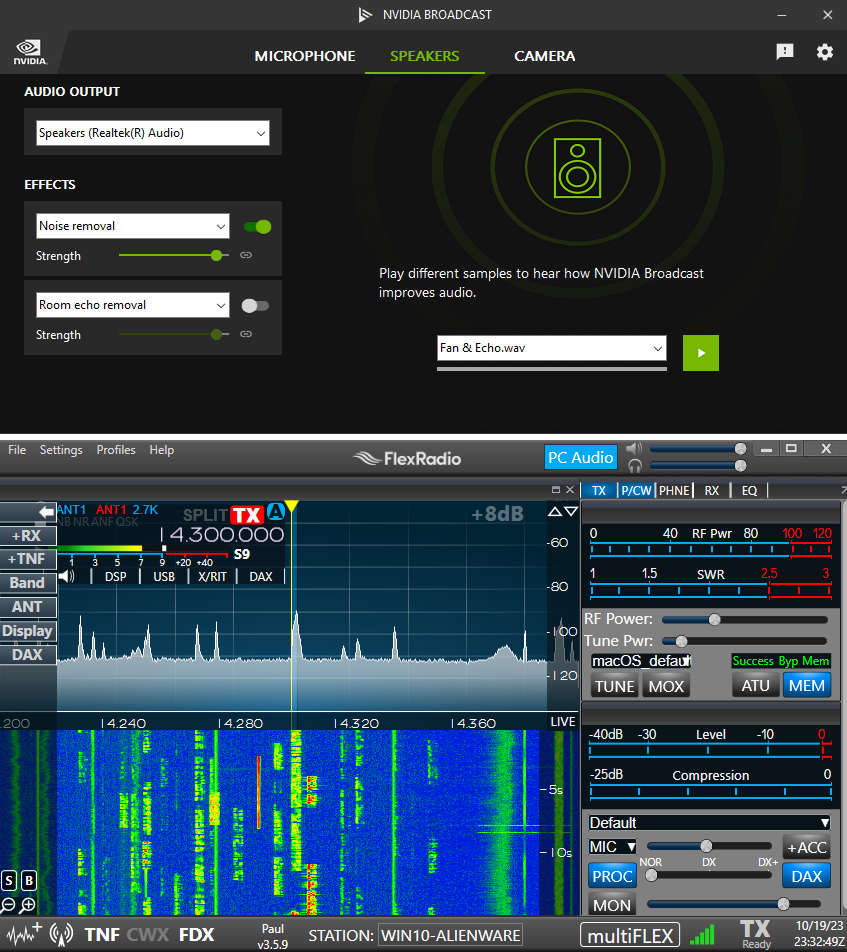
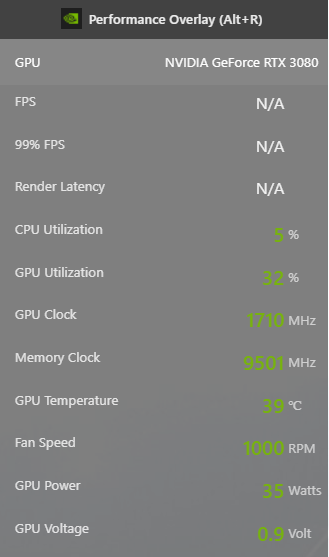
AI Runtime
RM Noise is a cloud-based application that uses a Windows client program to send and receive audio. The only hardware requirements are: “A Windows computer with internet access and the audio line in connected to a radio” according to their website.
The noisy audio from the radio is sent to a RM Noise dedicated inference server over the Internet for noise reduction. Besides returning the AI-cleaned audio to the end user in real-time, the audio could be retained to improve the AI model. As more people use the model, the data scientist has more audio to improve the customized noise reduction AI model.
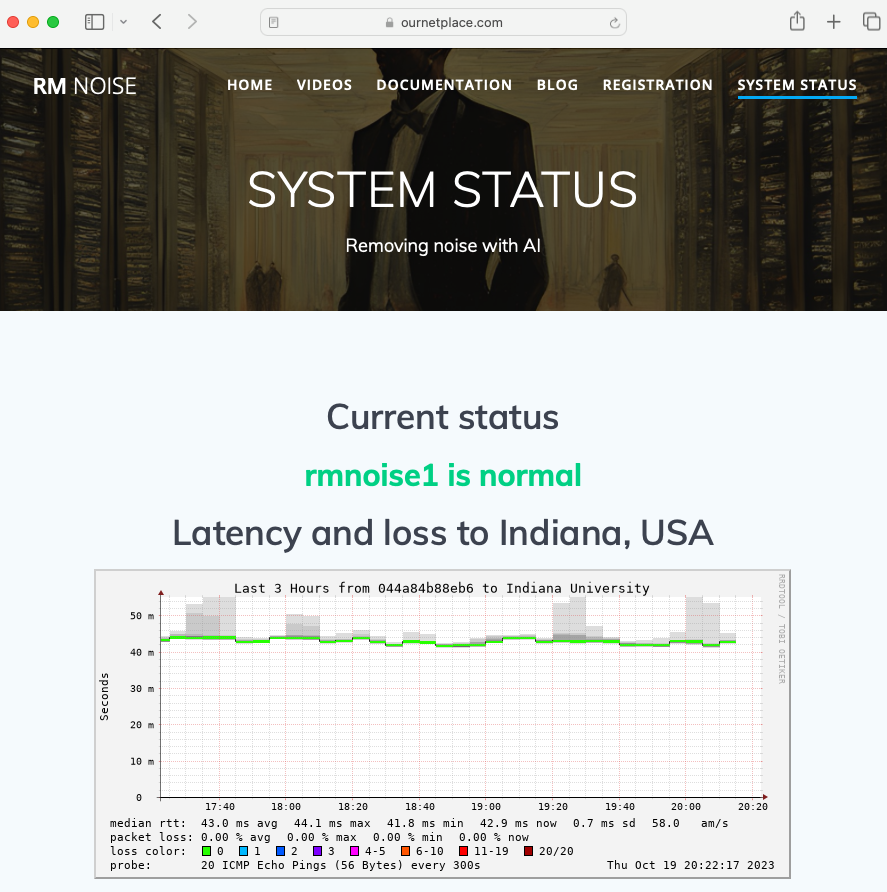
AI Model Demystification – 40,000 foot view
AI deployment in Amateur Radio is bleeding edge and not understood by most operators. RM Noise uses an AI Model to make decisions with pattern recognition on what sounds are noise and need elimination. This core of RM Noise is a predictive model based on “listening” to a lot of amateur radio traffic.
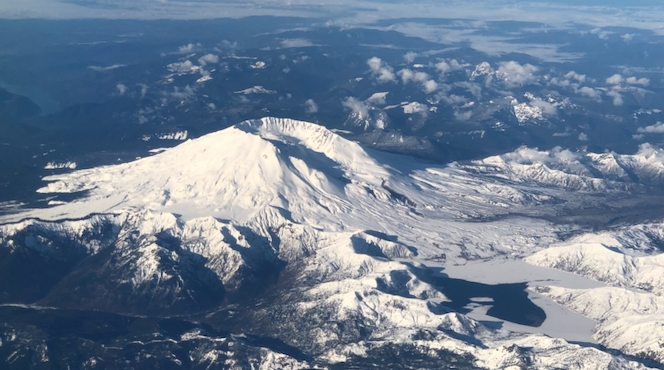
My description below is based on AI fundamentals I learned while working with Data Scientists at Microsoft developing Windows 10. The 40,000-foot view of the RM Noise AI platform includes data collection, predictive model development, runtime deployment in the cloud, and continuous improvement.
Data Collection: A LOT of typical amateur radio traffic, both phone and CW, is collected in digital form. The data requires curation. This curation includes preprocessing, structuring, and cleaning. They manually inspect every recording prior to including it in the training dataset. Various data are needed, examples include different propagation conditions, spoken languages, accents, radios, QRM, QRN, CW paddle, CW straight key, CW speeds, and bandwidths.
Predictive Model Development: Existing AI models and architectures are explored with the data collected. Experiments with statistical techniques are used to measure how accurately the model’s predictions match the desired noise reduction. If the experiment bombs, the data scientist will try a new model or architecture and validate that the data was curated correctly. Potentially, more diverse data is required. Regardless, many experiments are conducted to improve the model. This is how the model is trained with actual amateur radio audio traffic. I didn’t understand when I took statistics, and quantified business analysis classes would be prerequisites for understanding AI.
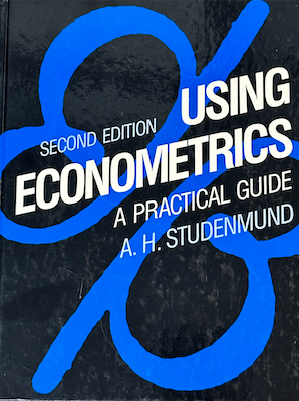
Cloud Runtime Deployment: Once the RM Noise data scientist is satisfied with the results, the AI model runtime, also known as an inference server, is deployed on the Internet. Amateur Radio operators with the RM Noise Python-based Windows PC program will send all of their radio audio to the inference server on the Internet, returning cleaned audio.
Continuous Improvement: RM Noise accepts audio recordings for tests and problems. This data goes back to all of the previous steps already described to include in the model. The noise reduction service continually improves over time.
Whats Next?
Amateur Radio AI noise elimination is at the beginning of the change adoption curve. Innovators are starting to use it and enjoy the value it brings. There is also a need for local AI noise elimination for Amateur Radio field day and other places where radio operators don’t have Internet access. I expect these AI models to become embedded in local processing accessories and embedded inside the radio. I’m glad I took the time to investigate the RM noise, the most significant innovation in Amateur Radio this year I’ve experienced.